Invited Speaker
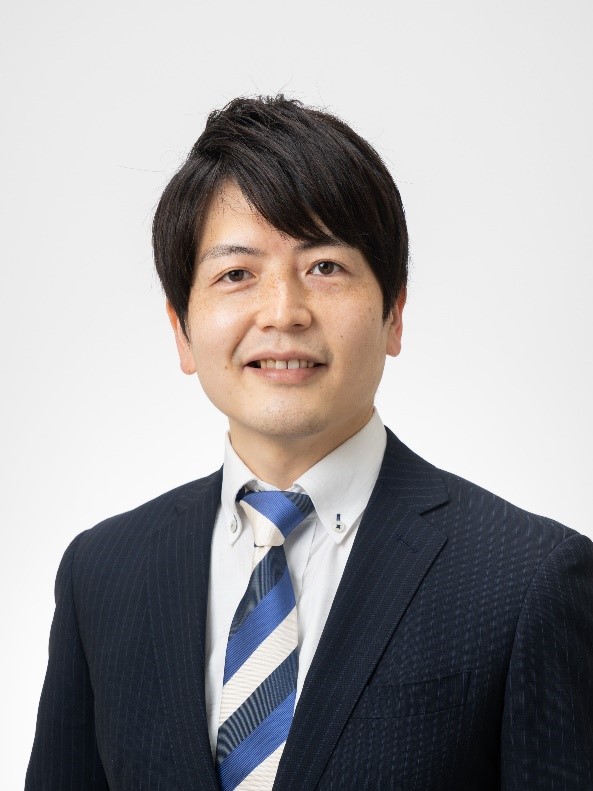
Assoc. Prof. Takuji Matsumoto
Faculty of Transdisciplinary Sciences for Innovation, Kanazawa University, JapanSpeech Title: Construction of Interpretable GAM-Based PV Forecasts That Outperform the Prediction Accuracy of Machine Learning Methods
Abstract: In recent years, the rapid increase in photovoltaic (PV) power generation worldwide has increased the need for a highly accurate PV power generation forecasting model that is easy to implement for a wide range of electric utilities. Against this background, this study proposes PV generation forecasting models based on the generalized additive model (GAM) and examines their properties from several perspectives, including ease of interpretation and comparison of forecast accuracy with multiple machine learning methods. Specifically, we construct different GAM-based forecast models with respect to regional and individual PV power generations in Japan and confirm the effectiveness of the models by visualizing the estimated trends for their intuitive and rational interpretations. In particular, for the individual PV generation model, a new forecasting model using a 3D tensor product spline is constructed, and it is shown that the robustness and prediction accuracy of the proposed model can be improved by incorporating smoothing conditions in three different directions of season, time, and solar radiation. The prediction performance of each GAM-based model is also compared with popular machine learning (ML) methods such as the k-nearest neighbor, artificial neural networks, support vector regression, and random forests. As a result, the GAM-based models are found to outperform these ML methods in terms of both computational efficiency and prediction accuracy, where the superiority of GAM-based models in prediction accuracy is especially evident when complementing periodic trends. Our empirical results suggest that the GAM-based models, a statistical approach that more easily reflects the modeler's prior knowledge, facilitate more rational and robust model building than the ML methods, which rely more on pattern recognition of data, and can therefore contribute to improving prediction accuracy.